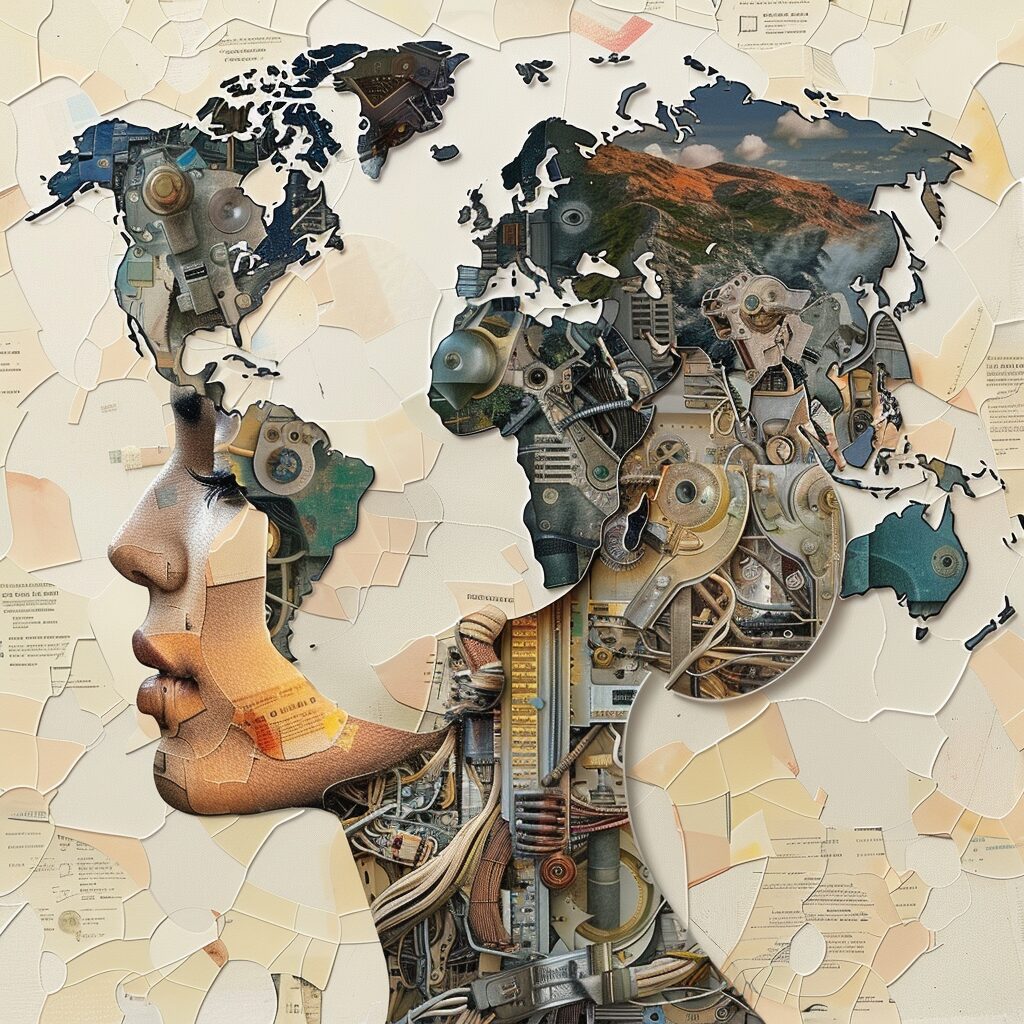
Like most of the tech world, we at Accion Venture Lab have been learning about and experimenting with generative AI (GenAI) over the past year. While there is still much to learn, we remain excited about the potential applications of GenAI for financial inclusion. Artificial intelligence applications in inclusive fintech are not new by any means; the past decade has seen use cases in fraud detection, underwriting, risk pricing, and more. Many of our portfolio companies already leverage AI. Yet, the challenges of reaching and serving low-income and underserved populations — and doing so profitably and at scale — persist. We believe generative AI advancements that complement current AI use cases can address these financial inclusion challenges head-on.
Over the past year, we’ve documented and categorized the opportunities we see in AI for financial inclusion into internal use cases (i.e., within the organization) and external use cases (i.e., interactions with customers, suppliers, regulators, and others). We believe GenAI has the potential to address both for a financial institution by either automating the previously inefficient workflows or serving as a co-pilot that can advise or support current staff in executing a workflow more efficiently. The former is appropriate for procedural and repetitive tasks, while the latter is suitable for workflows where trust in digital interactions is low or a high cost of failure means that financial institutions prefer human involvement. With these considerations, we built this framework to pinpoint AI’s role in overcoming financial inclusion challenges.
Identifying pressing challenges of financial inclusion that can be solved by AI
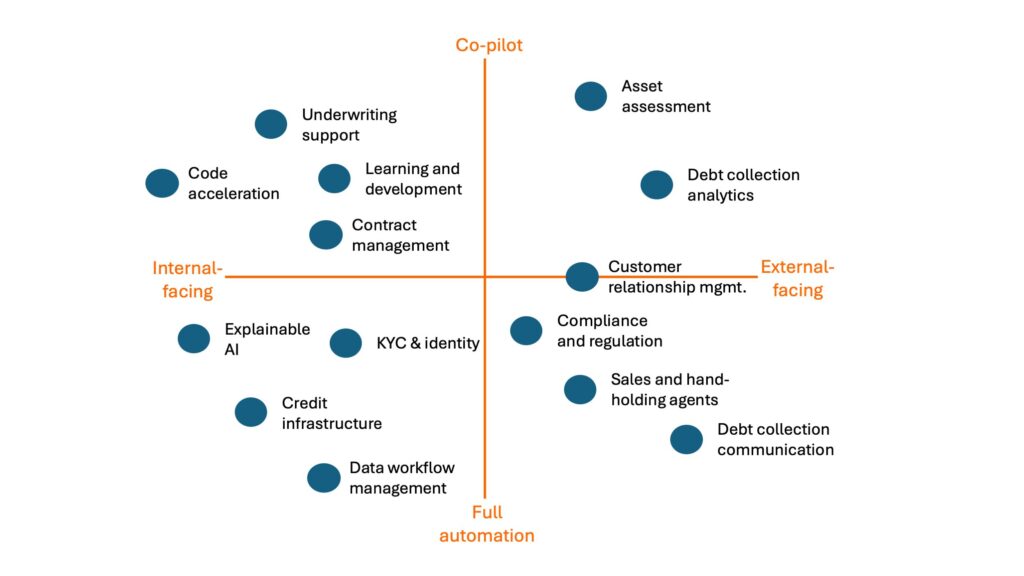
From this landscape of opportunities, we are currently excited about GenAI’s potential to solve challenges in these categories:
1. Bringing down the cost of KYC and compliance for hard-to-reach customers
For fintechs in frontier markets, the cost of Know Your Customer (KYC) can be prohibitive. Contributing costs can include handholding for new-to-digital customers, cross-verifying sub-optimal data sources, conducting manual checks, handling paper trails, and high rejection rates. We are interested in GenAI-powered solutions that lower costs and increase reach for onboarding workflows like KYC. Use cases include:
- GenAI-powered automated document verification and natural language processing models to extract KYC-relevant data from large troves of unstructured data, thus reducing cost. For example, Caf in Brazil analyzes a combination of data points, such as facial biometrics and document verification, to establish accurate identification.
- Full-stack onboarding solutions, like Idfy, offer regulation-compliant AI features, such as bandwidth-adjusted video KYC, that enable financial service providers to onboard customers from remote locations.
2. Underwriting thin-file and no-file borrowers more accurately
Successfully underwriting customers without a data footprint requires more than an accurate underwriting model. A successful underwriting function requires accurate and efficient data collection and the ability of risk officers to interpret model output and make decisions. GenAI can optimize and improve credit decisioning by streamlining how teams manage and process data.
Illustration of a full-stack* AI-led credit infrastructure for thin-file customers
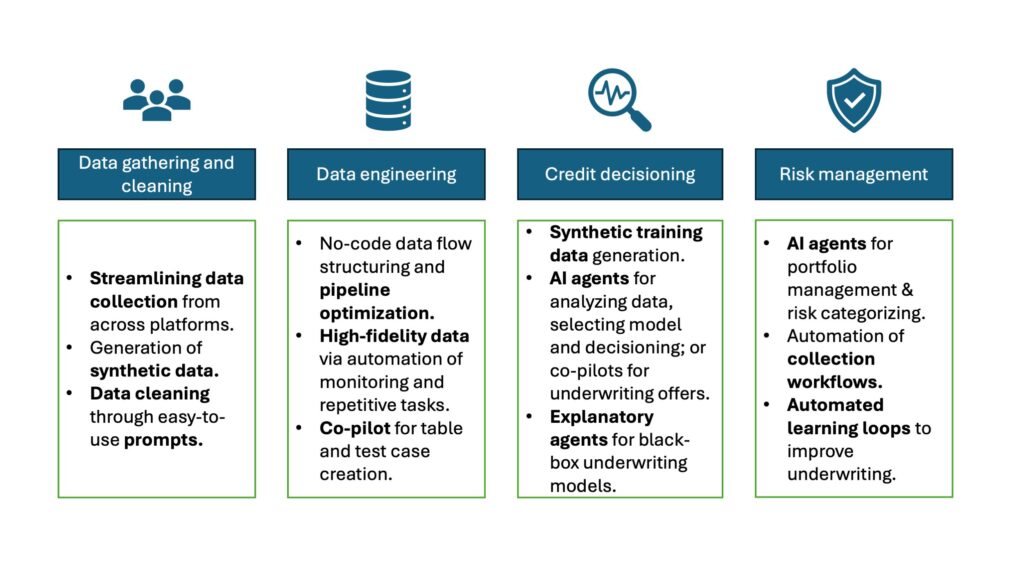
- Underwriting starts with data collection by field staff. Data gathering for small-business loans, such as taking stock of inventory, plant machinery, and farm acreage, can get complex. GenAI can be used to take in inputs like images, video, and speech and contextually prompt field staff to collect data and help make decisions, boosting productivity and accuracy while lowering costs. While we have not seen companies building specifically for this use case yet, we believe this is an untapped opportunity in end-to-end credit-decisioning infrastructure.
- GenAI products such as Mostly.ai can improve the predictive power of underwriting models by generating synthetic training datasets. A verticalized focus on generating synthetic data to train underwriting models can complement the data of thin-file customers by expanding the training set.
- Dvara Solutions is prototyping a co-pilot for underwriting officers, enhancing decision-making efficiency. We believe that using such co-pilot products to upskill and unlock efficiencies internally represents a valuable whitespace where solutions can be built by new AI-native entrants or existing vertical software.
- Underwriting thin-file customers with volumes of unstructured data requires complex models such as deep neural networks. Such models can be accurate but are opaque. When serving thin-file customers, it is critical to understand the rationale behind decisions since a mistake in the model can deny access to deserving customers. Explainable AI (XAI), when deployed with predictive AI in underwriting models, can provide transparency which aids risk teams in course corrections. GenAI can layer above such XAI models to provide easily consumable model explanations.
3. Engaging with customers in a more meaningful and cost-efficient way
Users on embedded finance platforms face a big leap from analog to digital transactions, requiring costly handholding. GenAI can provide support for product discovery, customer support, and debt collection for companies offering lending. Examples include:
- Leveraging custom language generators as agents can contextualize and assist users. Sukhiba uses generative AI for conversational commerce, supporting the salesforce in existing distributor supply chains and packaged consumer goods companies by guiding retailers, wholesalers, and consumers in placing orders, even creating AI-generated shopping carts based on their needs.
- AI can optimize customer support functions with automated GenAI holding conversations tailored to new-to-digital customers for procedural tasks like onboarding. GenAI in a co-pilot context can assist customer support agents in catering to high-value and complex flows, such as understanding the needs of a small business through AI-generated nudges and context on customers.
- Effective debt collection hinges on relationship management and communication strategies. Generative AI can layer empathy and personalization to communications, setting AI-led collections apart from traditional, often aggressive, approaches. Through prediction, automation, and generation, companies like DPDZero in India and Pallav in Indonesia are leveraging AI to enable full-stack debt collection through risk-based borrower categorization and tailored communication.
4. Helping fintechs optimize internal workflows
With workers becoming increasingly comfortable with digital interfaces and GenAI use gaining steam in workplaces, we think applications of GenAI that allow workers to perform internal tasks more efficiently can enable startups to pass on more value to underserved customers. In many cases, such efficiencies can also help frontier fintechs become sustainable in the long run, such as:
- Businesses with field staff face high turnover in such teams, leading to high hiring and retraining costs. Tools like Dvara’s Sarathi use generative AI onboarding agents on mobile devices to cut ramp-up time, save costs, and free up management bandwidth.
- Financial providers in emerging markets increasingly need reliable partners for their data journey, from data sourcing to embedding AI. Moffin aids data-driven financial institutions with AI-ready infrastructure, expanding data sources and improving internal workflows. We are excited about software products that accelerate the AI journey of FSPs, which, in turn, helps deepen financial inclusion.
While the opportunities are abundant, and we are excited about the potential for AI to accelerate inclusive fintech, founders should tread with caution as the risks from a faulty model without checks can outweigh the potential gains. At Accion Venture Lab, we are committed to championing equitable AI, where technology not only innovates but also accelerates our journey to fair and equitable financial inclusion.